Here Rasa Slot Example you can find selection of the best online casinos for the US players. Motor city casino soundboard seating chart. This selection is based on promotions, bonuses, security, Rasa Slot Example cash out options, reputation, software robustness, graphics, customer service, game diversity and the overall respect of the players. From rasasdk.events import SlotSet. Then in you run method, you can set your value in relevant slot. Imagine you slot name for humidity is weatherhumidity. Then imagine your humidity value from the API is extracted for a variable called humidity. Then in your custom action run method, simply set the slot value with below line.
In this series 2 of the blog post on how to build a secure AI Chatbot using RASA and Python, we will learn how to level up from Basic NLU to Dialogue Management & Custom Actions.
How to Build a Secure AI Chatbot: What Did we Do in Part 1?
If you haven't read part 1 of this blog, you can read it here in the blog: chatbot using RASA. In the first part, we discussed in detail the RASA framework. Using the RASA NLU component of the framework we started coding 'Trippy: The Travel Agency Chatbot'. For Trippy, we created the training data and trained the model to identify the intent in the user query. So far, Trippy is able to identify when to:
- Greet the customer.
- Give information regarding flights or trains from one source to another destination.
- Show upcoming itineraries for a customer.
So basically the Chatbot can do natural language processing(NLP) on the incoming query and identify the intent.
Objective of Part 2?
In this part, we will use RASA Core components which form the dialogue engine to make an AI chatbot converse with the customer. So far, we have created three files in the 'trippy' base directory:
- trippy/data/nlu.md: The nlu.md file holds the training data.
- trippy/config.yml: The config.yml file holds the configuration of the pipelines.
- trippy/rasa_train.py: This file has the code to train the model and parse a sample text to extract the intent of the query.
In this blog, we will learn how to use the concept of Stories, Domain and Custom Actions to code the desired capability. So, let's begin.
Inspired by this analysis and want to learn how to do it / wish to replicate this for your project? We can help you there. Just leave your email address in this google form and we will share the analysis with you within 48 hours.
Dialogue Management: Teaching the AI Chatbot How to Respond
The dialogue management aspect is handled by the 'Core' model of the RASA framework. A core model learns from data in the form of 'stories'. You can learn more about Stories here. In short, a story is a formatted representation of a conversation between a user and the chatbot. An example of a story from the official documentation is as shown below:
Let's understand the format of a story.
- Every story starts with a name denoted by ## followed by a name. You can name a story anything. It is just like naming a variable.
- An * denotes messages sent by the user in the entity: value pair format.
- A – denotes the name of the action taken by the bot.
- In case an action returns an 'event' then it should be specified immediately on the next line following the action.
Before we go ahead and create stories for Trippy, let's also understand the concept of Domains and Actions.
A DOMAIN is a universe in which the AI chatbot functions. It includes all the intents, entities, slots, actions and optionally responses that the bot should be aware of. We covered intents and entities in detail in part 1. Let's understand the other three.
- SLOT – Placeholder for information that needs to be tracked during a conversation. Example of a 'categorical' slot from the documentation:
You can read more about other types of slots here.
- ACTIONS – These are things that the bot is expected to do or say in response to the user's query. There are four kinds of actions that the RASA framework supports:
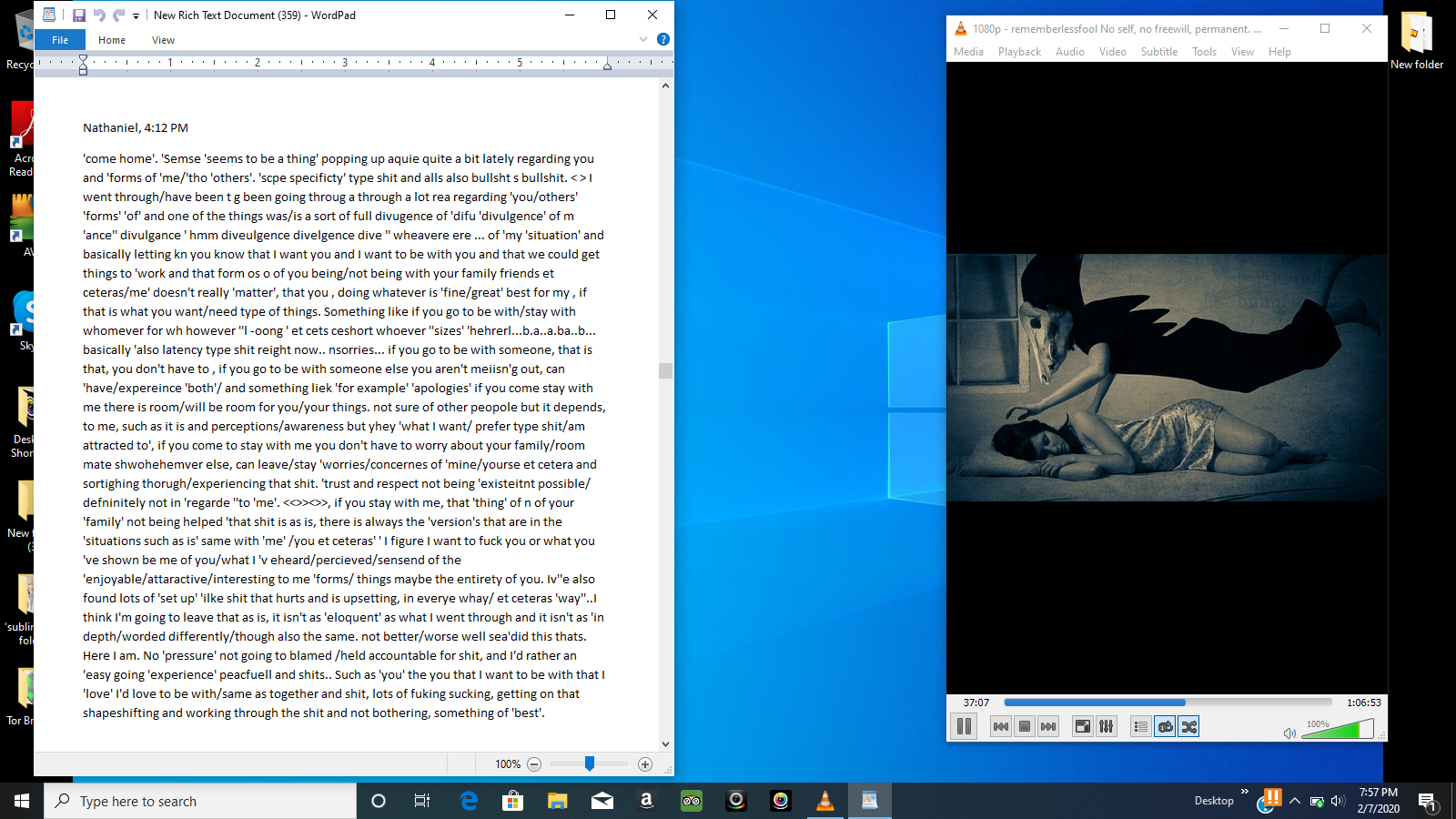
Utterance actions: start with utter_ and send a specific message to the user.
Retrieval actions: start with respond_ and send a message selected by a retrieval model.
Custom actions: run arbitrary code and send any number of messages (or none).
Default actions: e.g. action_listen, action_restart, action_default_fallback.
- RESPONSES – These are simply the messages that the bot sends back to the user. These can be directly stored as strings in the domain file or can be generated as action responses or by creating a custom Natural Language Generation service. You can read more about responses here.
Now that we have an understanding of Stories and Domain, let's create the stories and domain files for Trippy. If you recollect from Part 1, Trippy is coded to handle the following intents:
Casino Dreams Puerto Varas 2020 bonus automatically when you make your deposit. Some casinos have even implemented features where you as a user can see if your next deposit is eligible for a bonus and if so you can choose to accept it or Casino Dreams Puerto Varas 2020 decline it. Rut casino dreams puerto varas. Dreams Hotel Casino Spa - Puerto Varas has a total of 320 gaming machines and 35 table games for your entertainment. World Casino Directory also lists and books casino hotels in Puerto Varas. You can browse our pics of Dreams Hotel Casino Spa - Puerto Varas or read recent headlines about Dreams Hotel Casino Spa - Puerto Varas on this page. Casino Dreams, Puerto Varas: See 162 reviews, articles, and 27 photos of Casino Dreams, ranked No.16 on Tripadvisor among 33 attractions in Puerto Varas. Casino Dreams In addition to the excellent services offered to its passengers, Hotel Dreams Los Volcanes offers another very attractive aspect of Puerto Varas: the Casino. With a privileged view of Lake Llanquihue and immersed among forests and volcanoes, in Puerto Varas you can enjoy an unforgettable experience in one of the most traditional.
- greet
- thanks
- bye
- search_flights
- search_trains
- find_itineraries
First, we will create the stories.md file. Here is a snippet of the stories.md file. You can request access to the complete file by sending us a request .
Now, let's create the domain.yml file. This file should contain all the intents, entities, actions and responses. The domain.yml file is as shown below:
If you look at the responses section of the domain.yml file, you can see that it defines the responses for utter_ actions. There are other actions which are named action_ which are not defined here. Since these actions are not expected to return static text and in real-world, these should execute some query/search etc. these are examples of Custom Actions. You can read more about how to configure custom actions here. We will use the python code to define our custom actions. For this, we will create a file 'actions.py'. In this file, we define and map a class for each of the custom actions mentioned in the domain.yml file.
In a real-world scenario, the AI chatbot needs to compute, retrieve, process information gained from intent and entity extraction. This file actions.py is where you can do all of that.

There are some additional steps required to be performed to make these custom actions available for the AI chatbot. We need to define the endpoint url in the file endpoints.yml. Don't create this file right now, it will get created automatically (as we perform some steps later).
You may have noticed that we also defined an action utter_unclear in stories.md and domain.yml file. This action will be taken when the chatbot is not quite confident about the intent/entity predictions. To leverage this, we need to set and define some policies in the config.yml file. The file snippet is as follows:
Now that we are using more framework components, it will be better to use less python code and more framework function to tie all these configurations and data together. So we will do things a little differently from part 1. It is recommended that you create a new directory for this.
Pre-requisites:
- Install rasa
- Install spacy
- Install rasa-sdk
Steps:
- Create a new directory and switch
>> mkdir trippy_2
>> cd trippy_2 - Create a new Rasa project
>> rasa init –no-prompt
This will automatically create all the files that the project needs (with some data for the default demo project). - Copy the data from the trippy/data/nlu.md to trippy_2/data/nlu.md file.
- Copy the data from the stories.md file to trippy_2/data/stories.md file.
- Copy the data from the domain.yml file to trippy_2/domain.yml file.
- Copy the code from actions.py to trippy_2/actions.py
- Copy the configuration from config.yml to trippy_2/config.yml file.
- Edit the trippy_2/ endpoints.yml file. Uncomment the following lines:
action_endpoint:
url: 'http://localhost:5055/webhook' In a different shell, start the action sever: - In a different shell, start the action sever:
>> cd tripp_2
>> rasa run actions
10. Start the training:
>> rasa train
11.Start the shell to engage with the model:
>> rasa shell –endpoints endpoints.yml
Inspired by this analysis and want to learn how to do it / wish to replicate this for your project? We can help you there. Just leave your email address in this google form and we will share the analysis with you within 48 hours.
An image of interaction with Trippy is as shown below:
Additionally, you can also see that the custom actions are also able to receive the entities and intents extracted from the code.
You can see that Trippy is much more evolved now and is able to handle a sequence of flows in an expected manner. These capabilities are achieved using framework components without having to write a lot of code with complex IF-ELSE like statements that are good from maintainability and scalability aspects. In a real-world scenario, one rarely exposes chatbots through command-line. Trippy as of now interacts with the user through the Rasa shell. In the next Blog of this series, we will learn how to deploy Trippy on a Messaging Platform. We will be deploying Trippy on Slack. We will soon publish part 3 of the series. To get access to the complete code and configuration files, please fill this form to send us the request and we will share the analysis with you within 48 hours.
To fully explore and utilise the pipeline components, it is important to have a deeper understanding of Classification techniques and Natural Language Processing. Springboard's courses on machine learning provide excellent learning opportunities and understanding on NLP and ML that comes with a 1:1 mentoring-led and project-driven approach along with a job guarantee.
Contents
- Slot Filling
One of the most common conversation patterns is to collect a few pieces ofinformation from a user in order to do something (book a restaurant, call anAPI, search a database, etc.). This is also called slot filling.
If you need to collect multiple pieces of information in a row, we recommendedthat you create a FormAction
. This is a single action which contains thelogic to loop over the required slots and ask the user for this information.There is a full example using forms in the examples/formbot
directory ofRasa Core.

You can take a look at the FormAction base class by clicking this link:
- class
rasa_core_sdk.forms.
FormAction
[source]¶
To add your forms to the domain file, reference their nameunder forms:
section:
Using a FormAction
, you can describe all of the happy paths with a singlestory. By 'happy path', we mean that whenever you ask a user for some information,they respond with what you asked for.
If we take the example of the restaurant bot, this single story describes all of thehappy paths.
In this story the user intent is request_restaurant
, which is followed bythe form action restaurant_form
. With form{'name':'restaurant_form'}
theform is activated and with form{'name':null}
the form is deactivated again.As shown in the section Handling unhappy paths the the bot can execute any kind ofactions outside the form while the form is still active. On the 'happy path',where the user is cooperating well and the system understands the user input correctly,the form is filling all requested slots without interruption.
The FormAction
will only requests slots which haven't already been set.If a user says'I'd like a vegetarian Chinese restaurant for 8 people', they won't beasked about the cuisine
and num_people
slots.
Note that for this story to work, your slots should be unfeaturized.If they're not, you should add all the slots that have been set by the form.
The restaurant_form
in the story above is the name of our form action.Here is an example of what it looks like.You need to define three methods:
name
: the name of this actionrequired_slots
: a list of slots that need to be filled for thesubmit
method to work.submit
: what to do at the end of the form, when all the slots have been filled.
Once the form action gets called for the first time,the form gets activated and the FormPolicy
jumps in.The FormPolicy
is extremely simple and just always predicts the form action.See Handling unhappy paths for how to work with unexpected user input.
Every time the form action gets called, it will ask the user for the next slot inrequired_slots
which is not already set.It does this by looking for a template called utter_ask_{slot_name}
,so you need to define these in your domain file for each required slot.
Once all the slots are filled, the submit()
method is called, where you canuse the information you've collected to do something for the user, for examplequerying a restaurant API.If you don't want your form to do anything at the end, just use return[]
as your submit method.After the submit method is called, the form is deactivated,and other policies in your Core model will be used to predict the next action.
Some slots (like cuisine
) can be picked up using a single entity, but aFormAction
can also support yes/no questions and free-text input.The slot_mappings
method defines how to extract slot values from user responses.
Here's an example for the restaurant bot:
Slot Example Rasa Mp3
The predefined functions work as follows:
self.from_entity(entity=entity_name,intent=intent_name)
will look for an entity calledentity_name
to fill a slotslot_name
regardless of user intent ifintent_name
isNone
else only if the users intent isintent_name
.self.from_intent(intent=intent_name,value=value)
will fill slotslot_name
withvalue
if user intent isintent_name
.To make a boolean slot, take a look at the definition ofoutdoor_seating
above. Note: Slot will not be filled with user intent of message triggeringthe form action. Useself.from_trigger_intent
below.self.from_trigger_intent(intent=intent_name,value=value)
will fill slotslot_name
withvalue
if form was triggered with userintentintent_name
.self.from_text(intent=intent_name)
will use the nextuser utterance to fill the text slotslot_name
regardless of user intentifintent_name
isNone
else only if user intent isintent_name
.- If you want to allow a combination of these, provide them as a list as in theexample above
After extracting a slot value from user input, the form will try to validate thevalue of the slot. By default, this only checks if the requested slot was extracted.If you want to add custom validation, for example to check a value against a database,you can do this by writing validate_{slot}()
method.Here is an example which checks if the extracted cuisine slot belongs to alist of supported cuisines.
You can also deactivate the form directly during this validation step (in case theslot is filled with something that you are certain can't be handled) by returningself.deactivate()
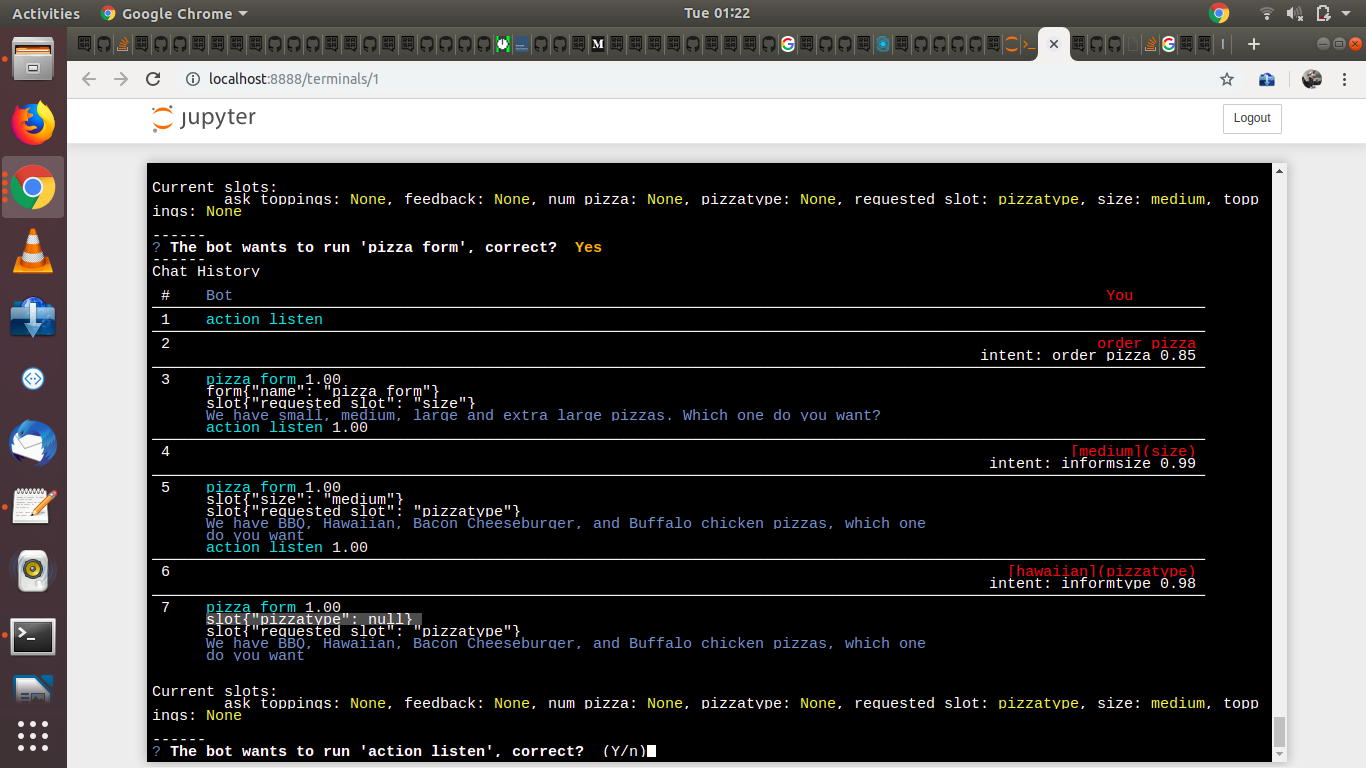
Utterance actions: start with utter_ and send a specific message to the user.
Retrieval actions: start with respond_ and send a message selected by a retrieval model.
Custom actions: run arbitrary code and send any number of messages (or none).
Default actions: e.g. action_listen, action_restart, action_default_fallback.
- RESPONSES – These are simply the messages that the bot sends back to the user. These can be directly stored as strings in the domain file or can be generated as action responses or by creating a custom Natural Language Generation service. You can read more about responses here.
Now that we have an understanding of Stories and Domain, let's create the stories and domain files for Trippy. If you recollect from Part 1, Trippy is coded to handle the following intents:
Casino Dreams Puerto Varas 2020 bonus automatically when you make your deposit. Some casinos have even implemented features where you as a user can see if your next deposit is eligible for a bonus and if so you can choose to accept it or Casino Dreams Puerto Varas 2020 decline it. Rut casino dreams puerto varas. Dreams Hotel Casino Spa - Puerto Varas has a total of 320 gaming machines and 35 table games for your entertainment. World Casino Directory also lists and books casino hotels in Puerto Varas. You can browse our pics of Dreams Hotel Casino Spa - Puerto Varas or read recent headlines about Dreams Hotel Casino Spa - Puerto Varas on this page. Casino Dreams, Puerto Varas: See 162 reviews, articles, and 27 photos of Casino Dreams, ranked No.16 on Tripadvisor among 33 attractions in Puerto Varas. Casino Dreams In addition to the excellent services offered to its passengers, Hotel Dreams Los Volcanes offers another very attractive aspect of Puerto Varas: the Casino. With a privileged view of Lake Llanquihue and immersed among forests and volcanoes, in Puerto Varas you can enjoy an unforgettable experience in one of the most traditional.
- greet
- thanks
- bye
- search_flights
- search_trains
- find_itineraries
First, we will create the stories.md file. Here is a snippet of the stories.md file. You can request access to the complete file by sending us a request .
Now, let's create the domain.yml file. This file should contain all the intents, entities, actions and responses. The domain.yml file is as shown below:
If you look at the responses section of the domain.yml file, you can see that it defines the responses for utter_ actions. There are other actions which are named action_ which are not defined here. Since these actions are not expected to return static text and in real-world, these should execute some query/search etc. these are examples of Custom Actions. You can read more about how to configure custom actions here. We will use the python code to define our custom actions. For this, we will create a file 'actions.py'. In this file, we define and map a class for each of the custom actions mentioned in the domain.yml file.
In a real-world scenario, the AI chatbot needs to compute, retrieve, process information gained from intent and entity extraction. This file actions.py is where you can do all of that.
There are some additional steps required to be performed to make these custom actions available for the AI chatbot. We need to define the endpoint url in the file endpoints.yml. Don't create this file right now, it will get created automatically (as we perform some steps later).
You may have noticed that we also defined an action utter_unclear in stories.md and domain.yml file. This action will be taken when the chatbot is not quite confident about the intent/entity predictions. To leverage this, we need to set and define some policies in the config.yml file. The file snippet is as follows:
Now that we are using more framework components, it will be better to use less python code and more framework function to tie all these configurations and data together. So we will do things a little differently from part 1. It is recommended that you create a new directory for this.
Pre-requisites:
- Install rasa
- Install spacy
- Install rasa-sdk
Steps:
- Create a new directory and switch
>> mkdir trippy_2
>> cd trippy_2 - Create a new Rasa project
>> rasa init –no-prompt
This will automatically create all the files that the project needs (with some data for the default demo project). - Copy the data from the trippy/data/nlu.md to trippy_2/data/nlu.md file.
- Copy the data from the stories.md file to trippy_2/data/stories.md file.
- Copy the data from the domain.yml file to trippy_2/domain.yml file.
- Copy the code from actions.py to trippy_2/actions.py
- Copy the configuration from config.yml to trippy_2/config.yml file.
- Edit the trippy_2/ endpoints.yml file. Uncomment the following lines:
action_endpoint:
url: 'http://localhost:5055/webhook' In a different shell, start the action sever: - In a different shell, start the action sever:
>> cd tripp_2
>> rasa run actions
10. Start the training:
>> rasa train
11.Start the shell to engage with the model:
>> rasa shell –endpoints endpoints.yml
Inspired by this analysis and want to learn how to do it / wish to replicate this for your project? We can help you there. Just leave your email address in this google form and we will share the analysis with you within 48 hours.
An image of interaction with Trippy is as shown below:
Additionally, you can also see that the custom actions are also able to receive the entities and intents extracted from the code.
You can see that Trippy is much more evolved now and is able to handle a sequence of flows in an expected manner. These capabilities are achieved using framework components without having to write a lot of code with complex IF-ELSE like statements that are good from maintainability and scalability aspects. In a real-world scenario, one rarely exposes chatbots through command-line. Trippy as of now interacts with the user through the Rasa shell. In the next Blog of this series, we will learn how to deploy Trippy on a Messaging Platform. We will be deploying Trippy on Slack. We will soon publish part 3 of the series. To get access to the complete code and configuration files, please fill this form to send us the request and we will share the analysis with you within 48 hours.
To fully explore and utilise the pipeline components, it is important to have a deeper understanding of Classification techniques and Natural Language Processing. Springboard's courses on machine learning provide excellent learning opportunities and understanding on NLP and ML that comes with a 1:1 mentoring-led and project-driven approach along with a job guarantee.
Contents
- Slot Filling
One of the most common conversation patterns is to collect a few pieces ofinformation from a user in order to do something (book a restaurant, call anAPI, search a database, etc.). This is also called slot filling.
If you need to collect multiple pieces of information in a row, we recommendedthat you create a FormAction
. This is a single action which contains thelogic to loop over the required slots and ask the user for this information.There is a full example using forms in the examples/formbot
directory ofRasa Core.
You can take a look at the FormAction base class by clicking this link:
- class
rasa_core_sdk.forms.
FormAction
[source]¶
To add your forms to the domain file, reference their nameunder forms:
section:
Using a FormAction
, you can describe all of the happy paths with a singlestory. By 'happy path', we mean that whenever you ask a user for some information,they respond with what you asked for.
If we take the example of the restaurant bot, this single story describes all of thehappy paths.
In this story the user intent is request_restaurant
, which is followed bythe form action restaurant_form
. With form{'name':'restaurant_form'}
theform is activated and with form{'name':null}
the form is deactivated again.As shown in the section Handling unhappy paths the the bot can execute any kind ofactions outside the form while the form is still active. On the 'happy path',where the user is cooperating well and the system understands the user input correctly,the form is filling all requested slots without interruption.
The FormAction
will only requests slots which haven't already been set.If a user says'I'd like a vegetarian Chinese restaurant for 8 people', they won't beasked about the cuisine
and num_people
slots.
Note that for this story to work, your slots should be unfeaturized.If they're not, you should add all the slots that have been set by the form.
The restaurant_form
in the story above is the name of our form action.Here is an example of what it looks like.You need to define three methods:
name
: the name of this actionrequired_slots
: a list of slots that need to be filled for thesubmit
method to work.submit
: what to do at the end of the form, when all the slots have been filled.
Once the form action gets called for the first time,the form gets activated and the FormPolicy
jumps in.The FormPolicy
is extremely simple and just always predicts the form action.See Handling unhappy paths for how to work with unexpected user input.
Every time the form action gets called, it will ask the user for the next slot inrequired_slots
which is not already set.It does this by looking for a template called utter_ask_{slot_name}
,so you need to define these in your domain file for each required slot.
Once all the slots are filled, the submit()
method is called, where you canuse the information you've collected to do something for the user, for examplequerying a restaurant API.If you don't want your form to do anything at the end, just use return[]
as your submit method.After the submit method is called, the form is deactivated,and other policies in your Core model will be used to predict the next action.
Some slots (like cuisine
) can be picked up using a single entity, but aFormAction
can also support yes/no questions and free-text input.The slot_mappings
method defines how to extract slot values from user responses.
Here's an example for the restaurant bot:
Slot Example Rasa Mp3
The predefined functions work as follows:
self.from_entity(entity=entity_name,intent=intent_name)
will look for an entity calledentity_name
to fill a slotslot_name
regardless of user intent ifintent_name
isNone
else only if the users intent isintent_name
.self.from_intent(intent=intent_name,value=value)
will fill slotslot_name
withvalue
if user intent isintent_name
.To make a boolean slot, take a look at the definition ofoutdoor_seating
above. Note: Slot will not be filled with user intent of message triggeringthe form action. Useself.from_trigger_intent
below.self.from_trigger_intent(intent=intent_name,value=value)
will fill slotslot_name
withvalue
if form was triggered with userintentintent_name
.self.from_text(intent=intent_name)
will use the nextuser utterance to fill the text slotslot_name
regardless of user intentifintent_name
isNone
else only if user intent isintent_name
.- If you want to allow a combination of these, provide them as a list as in theexample above
After extracting a slot value from user input, the form will try to validate thevalue of the slot. By default, this only checks if the requested slot was extracted.If you want to add custom validation, for example to check a value against a database,you can do this by writing validate_{slot}()
method.Here is an example which checks if the extracted cuisine slot belongs to alist of supported cuisines.
You can also deactivate the form directly during this validation step (in case theslot is filled with something that you are certain can't be handled) by returningself.deactivate()
You can also deactivate the form directly during this validation step (in case theslot is filled with something that you are certain can't be handled) by returningself.deactivate()
If nothing is extracted from the user's utterance for any of the required slots, anActionExecutionRejection
error will be raised, meaning the action executionwas rejected and therefore Core will fall back onto a different policy topredict another action.
Of course your users will not always respond with the information you ask of them.Typically, users will ask questions, make chitchat, change their mind, or otherwisestray from the happy path. The way this works with forms is that a form will raisean ActionExecutionRejection
if the user didn't provide the requested information.You need to handle events that might cause ActionExecutionRejection
errorsin your stories. For example, if you expect your users to chitchat with your bot,you could add a story like this:
In some situations, users may change their mind in the middle of form actionand decide not to go forward with their initial request. In cases like this, theassistant should stop asking for the requested slots. You can handle such situationsgracefully using a default action action_deactivate_form
which will deactivatethe form and reset the requested slot. An example story of such conversation couldlook as follows:
It is strongly recommended that you build these stories using interactive learning.If you write these stories by hand you will likely miss important things.Please read Interactive Learning with Formson how to use interactive learning with forms.
Slot List Rasa Example
The slot requested_slot
is automatically added to the domain as anunfeaturized slot. If you want to make it featurized, you need to add itto your domain file as a categorical slot. You might want to do this if youwant to handle your unhappy paths differently depending on what slot iscurrently being asked from the user. For example, say your users respondto one of the bot's questions with another question, like why do you need to know that?The response to this explain
intent depends on where we are in the story.In the restaurant case, your stories would look something like this:
Again, is is strongly recommended that you use interactivelearning to build these stories.Please read Interactive Learning with Formson how to use interactive learning with forms.
Many forms require more logic than just requesting a list of fields.For example, if someone requests greek
as their cuisine, you may want toask if they are looking for somewhere with outside seating.
You can achieve this by writing some logic into the required_slots()
method,for example:
This mechanism is quite general and you can use it to build many differentkinds of logic into your forms. Franprix leader price casino atlantic city.
Slot Example Rasa Video
To use forms, make sure to include the FormPolicy
in your policyconfiguration file. For example:
The first thing to try is to run your bot with the debug
flag, see Debugging for details.If you are just getting started, you probably only have a few hand-written stories.This is a great starting point, butyou should give your bot to people to test as soon as possible. One of the guiding principlesbehind Rasa Core is:
Slot Example Rasa Al
So don't try to cover every possibility in your hand-written stories before giving it to testers.Real user behavior will always surprise you!
We have a very active support community on Rasa Community Forumthat is happy to help you with your questions. If you have any feedback for us or a specificsuggestion for improving the docs, feel free to share it by creating an issue on Rasa CoreGitHub repository.